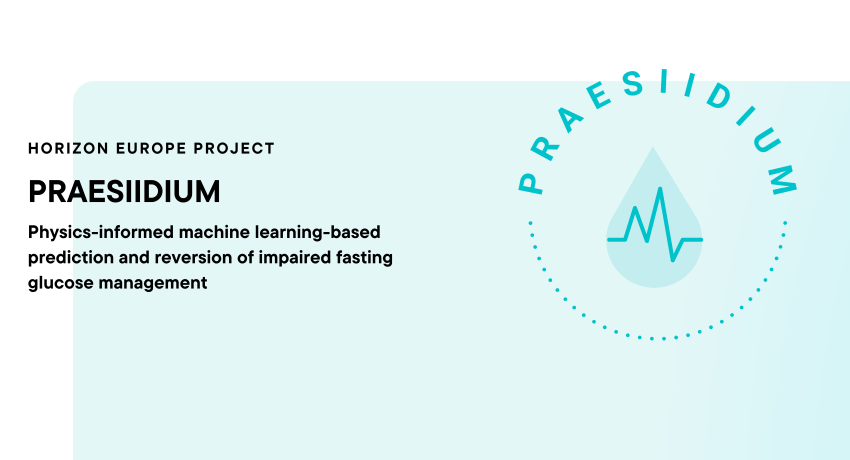
PRAESIIDIUM - Physics Informed Machine Learning-Based Prediction And Reversion Of Impaired Fasting Glucose Management
The incidence of undiagnosed diabetes accounts for 36% of European adults, while 541M adults worldwide have Impaired Glucose Tolerance (IGT), an important risk factor for further T2D development. Both IGT and/or Impaired Fasting Glucose (IFG) are intermediate glucose mishandling (i.e., intermediate conditions in the healthy-T2D transition) and are manifestations of the so-called prediabetes condition. Prediabetes itself is not an extensively studied condition compared to overt T2D, but it is also a condition that can be reversed without the prescription usage to not proceed into T2D. The aim of our project is to develop a prototype tool for the real-time prediction of the prediabetic risk based on a series of patient-specific mathematical models (firstly developed during the FP7 MISSION-T2D project) that simulate metabolism, pancreas hormone production, microbiome metabolites, inflammatory process, and immune system response. The prediction algorithm will be based on a “physics-informed machine learning” approach. A rich dataset of real-life data will be combined with a mathematical model to overcome the limits of a “black-box” ML approach, while reducing the computational time for simulating the solutions of heavy mathematical models and improving its prediction performances. We will collect the necessary training data (e.g., diet questionnaire, physical activity, blood metabolites, and microbiome) from already existing clinical studies (used as retrospective trials) which are representative of the real-life scenarios of a prediabetes/diabetes risk insurgence in adulthood (20–80y): family history, Metabolic Syndrome, Liver disease, and obesity. A newly dedicated multicentric pilot prospective observational study will also be performed, during which we will equip the participants with wearable sensors (e.g., glucose monitoring, bioimpedance, heart rate, accelerometer).