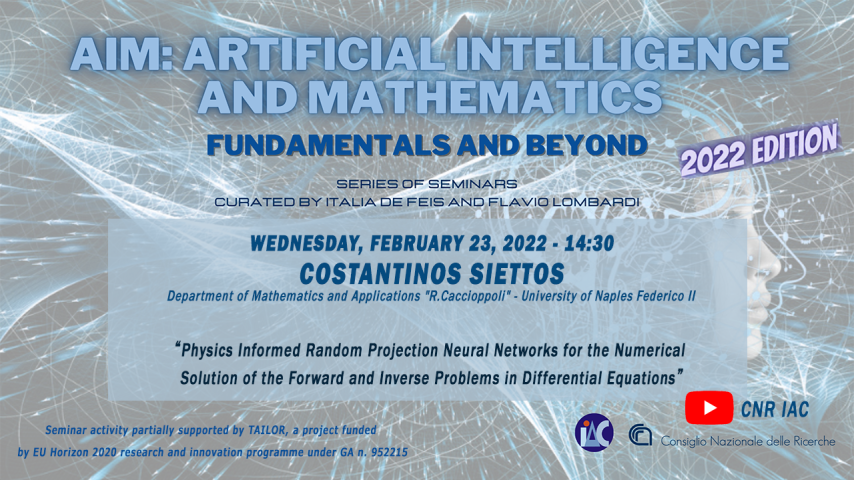
Over the last few years, machine learning has been used to solve both the forward, i.e. the numerical solution of time-depended non-linear differential equations as an alternative to classical numerical analysis methods, but also the inverse problem i.e. the problem of identifying and constructing models of differential equations.Here, I present how physics-informed Random Projection Neural Networks can be exploited to both numerically solve nonlinear PDEs and problems of ODEs with sharp gradients and high stiffness and identify the ``hidden" physics for construction of PDEs.Furthemore, I show how one can bridge this framework with the numerical bifurcation analysis toolkit to systematicalky study the complex dynamics. The performance of the proposed numerical method for the solution of the forwardproblem is assessed via several benchmark problems, and its efficiency is compared with other classical numerical methods including solvers for stiff ODES, Finite Differences and Finite Elements. For the solution of the inverse problem, I show how the proposed framerworkcan be efficiently used to to construct PDEs on a low-dimensional manifold based on large-scale spatio-temporal data. The proposed numerical framework is demontstated through the paradigm of Lattice Boltzmann simulations of the FitzHugh-Nagumo PDEs. The phsyics-informed RPNN scheme was quite effective in terms of numerical accuracy regarding the construction of the corresponding coarse-scale bifurcation diagram and 20 to 30 times less costly than the traditional shallow deep-learning scheme, thus arising as a promising alternative for solving both the foreward and inverse problems for high-dimensional PDEs.